Generative Science: Our Contrarian View of AI
Large science models will deliver transformative solutions in climate, biotech, and robotics
James Joaquin |
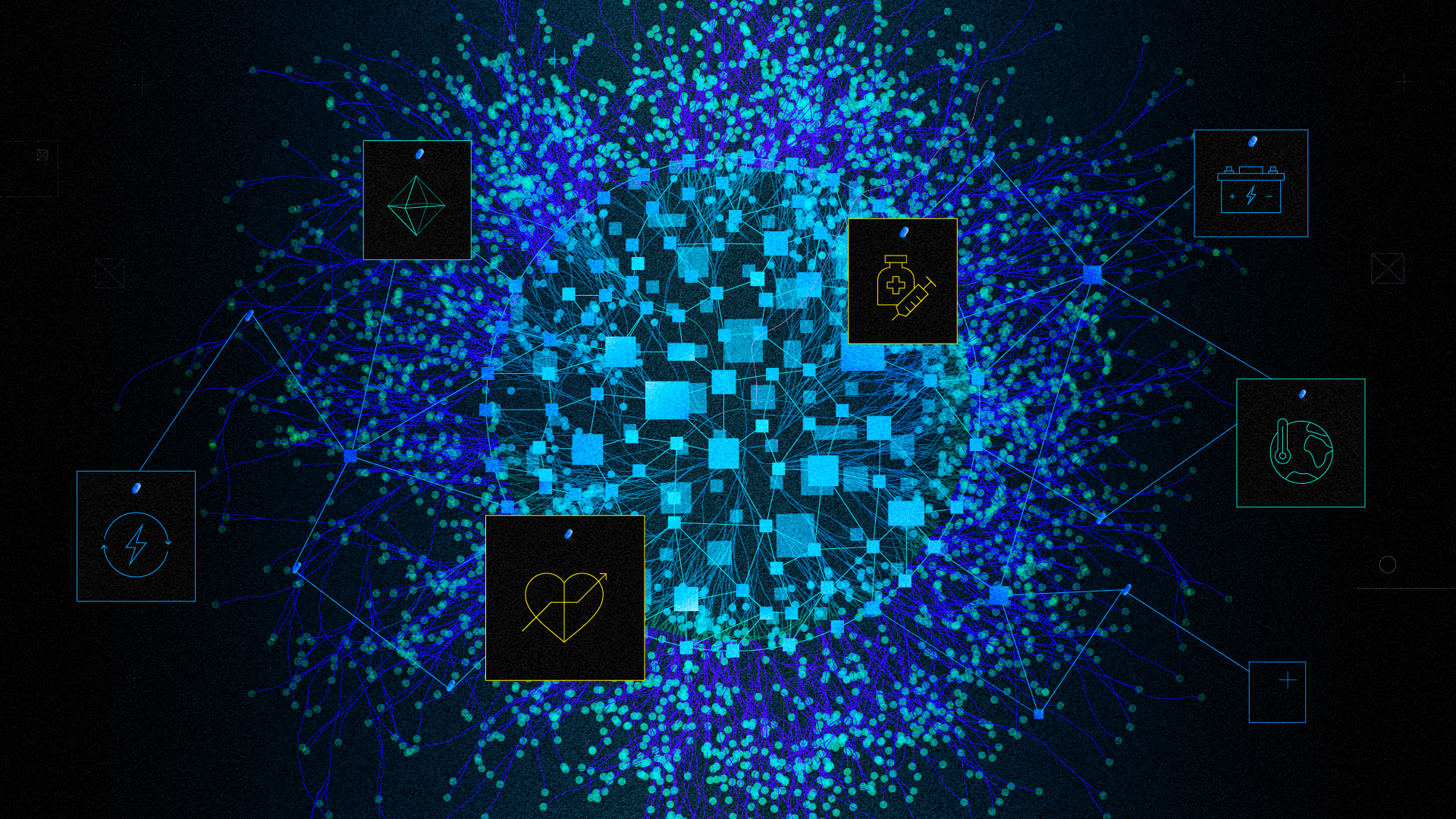
In 2017, eight researchers at Google published a groundbreaking paper called Attention is All You Need that laid the foundation for transformer-based artificial intelligence, or what we now know as generative AI. The first use of the technology was to generate media like text, images, and video.
Generative media has been a revolution. But the next wave of generative AI—generative science—will be far more transformative to the human race and usher in the next wave of trillion-dollar companies. While most venture dollars are chasing large language models for enterprise productivity, Obvious is funding large science models trained on chemistry, physics, and biology, to generate new scientific breakthroughs in decarbonization, biotech, materials science, and robotics.
The idea behind generative science is simple: train AI on our knowledge of the physical sciences like chemistry and biology, and then utilize that AI to generate scientific breakthroughs that discover new materials, new drugs, and new vaccines. Not only is generative science (or GenSci) already enabling breakthroughs, it’s also rewiring every field of science, from physics to chemistry to biology, with enormous potential for the future of our species and our planet.
We believe generative science will emerge as humanity’s greatest invention, because it is, for the first time, an invention that can invent.
Generative media has delivered us a printing press that can write its own words, but generative science will deliver a more consequential lab bench that can create its own novel arrangements of atoms. Those new chemicals and molecules will help solve humanity’s toughest challenges, from nuclear fusion to curing cancer, and open entirely new avenues of exploration that we’ve long considered beyond our reach, or have never considered at all.
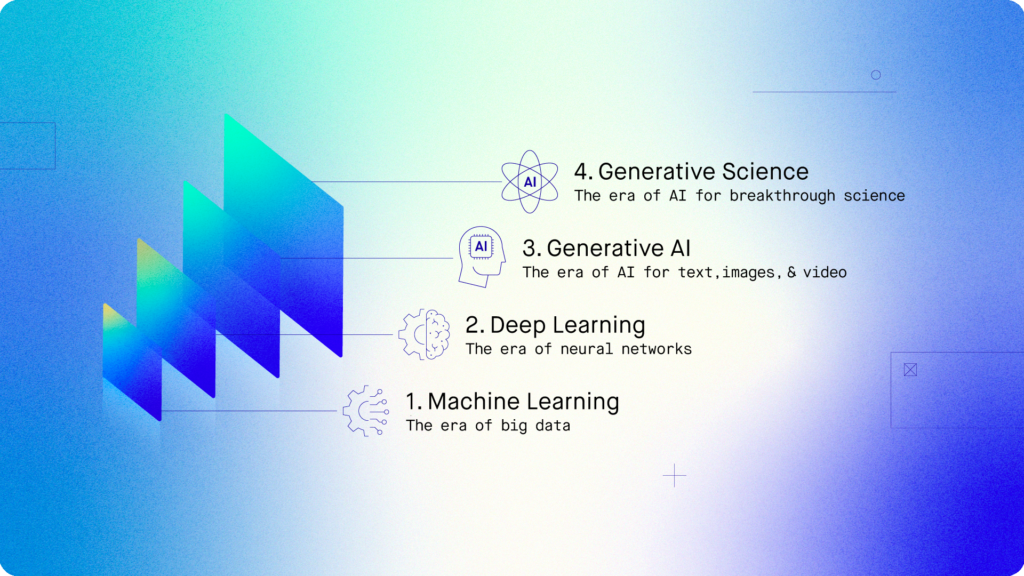
SOLVING THE UNSOLVABLE
Generative science can take on bigger quests with incredible speed. One of the world’s biggest obstacles over the next decade will be creating enormous new supplies of clean energy to power advances in AI, decarbonization, and the electrification of nearly every sector. By some estimates we need triple or quadruple our current baseload power.
Nuclear fusion is our best shot at unlimited clean energy, but it has remained out of reach for decades. Generative science is already enabling major progress much faster than human researchers possibly could. Using AI-powered learning systems, researchers in Switzerland have discovered how to control the extremely hot hydrogen plasma that collides in powerful fusion reactions, a crucial step that brings us closer than ever to a world-changing scientific breakthrough.
On another front, geothermal energy—long an expensive and hard to reach source of power—is also getting a major boost from generative science. The Utah-based geothermal company Zanskar has shown it can outperform the best human geologists in site exploration by applying AI to analyze geologic data to identify new energy sources and novel ways to reach them.
With rich new supplies of power, generative science will also help us store it in novel batteries. In a world running short of lithium, AI models are already discovering new battery chemistries. Last year, a Microsoft AI tool tasked with finding new battery consistencies to store and distribute energy took less than three days to narrow 32 million materials down to 18 and landed on a candidate that reduced lithium use by 70 percent.
On every front in our pursuit of a cleaner world, AI-assisted science is in the early stages of illuminating new technologies, improved methods, and novel materials. With each breakthrough, generative science incorporates its findings and builds upon them.
A NEW SCIENTIFIC METHOD
Traditional science has always worked on the old scientific method. It starts with a hypothesis about where to begin, followed by experiments, analysis, and a positive or negative conclusion. This method has brought us centuries of breakthroughs, from vaccines to anesthesia. But it’s constrained by the limits of human cognition and the slow pace of human analysis.
With generative science, this old method is going extinct. As we started to see in 2018, today’s models bring unprecedented capabilities in hypothesis generation, experimentation, and data analysis. Future iterations will autonomously generate vast quantities of data, simulate complex phenomena, and explore widespread possibilities across massive search spaces.
These systems will detect patterns, anomalies, and correlations in data that human researchers might otherwise never discover.
Biotechnology and healthcare are the richest spaces to witness the new scientific method in action. We already saw a major biotechnology revolution in 2020 when scientists used mRNA to develop Covid vaccines in record time. The technology behind the COVID vaccines that instructs cells to produce specific proteins can theoretically address almost any disease, including cancer, tuberculosis, HIV/AIDS, heart disease, and diabetes.
Scientists can’t find all of these curative pathways on their own. There are an impossibly large number of possible mRNA sequences—even a relatively simple protein like the spike protein in the Covid vaccines can be coded in as many as 2.4 x 10632 potential mRNA arrangements, a number exponentially greater than the 1082 atoms in the known universe. But well-trained generative science models can search across multi-dimensional data spaces and surface relevant pathways in unprecedented speed.
Biosoftware company Inceptive is leading the RNA revolution. Inceptive was founded in 2021 by Jakob Uszkoreit, one of the eight Google researchers who pioneered transformer-based AI. Inceptive’s generative technology is designed to discover new mRNA combinations to develop novel vaccines.
Similar innovation is already at play in other fields. Researchers have begun to use AI to predict protein structures, regulate the plasma inside a nuclear fusion reactor, and probe the size and structure of the entire universe based on the composition of one galaxy. And Google DeepMind just released a more powerful version of AlphaFold, an AI that can predict the behavior of microscopic mechanisms, including DNA and RNA, with the hope that this technology will accelerate the creation of new drugs.
ATOMS TO BITS, BITS TO ATOMS
The foundation of generative science uses biological, chemical, and physical data to train modern AI. The same way generative media analyzes millions of pieces of text and images to build creative replicas, generative science models are trained on DNA, RNA, nucleotides, protein amino acid sequences, cell images, chemical structure, and physical measurements.
Bridging these physical components with the digital world requires infrastructure that can convert chemical and biological matter (atoms) into data-rich information (bits) that can be processed and analyzed by computers. It must also act in reverse. Once the AI predicts a possible breakthrough—such as an mRNA molecule or protein sequence that can instruct cells to fight colorectal cancer—the data finding (expressed in bits) must be converted back into biological material (atoms) that can be tested, validated, and eventually deployed with high confidence of safety and efficacy into cancer patients.
New hardware and software needed for the advanced synthesis of chemicals and biological materials will be an exciting area of progress (and venture investment) in the years ahead.
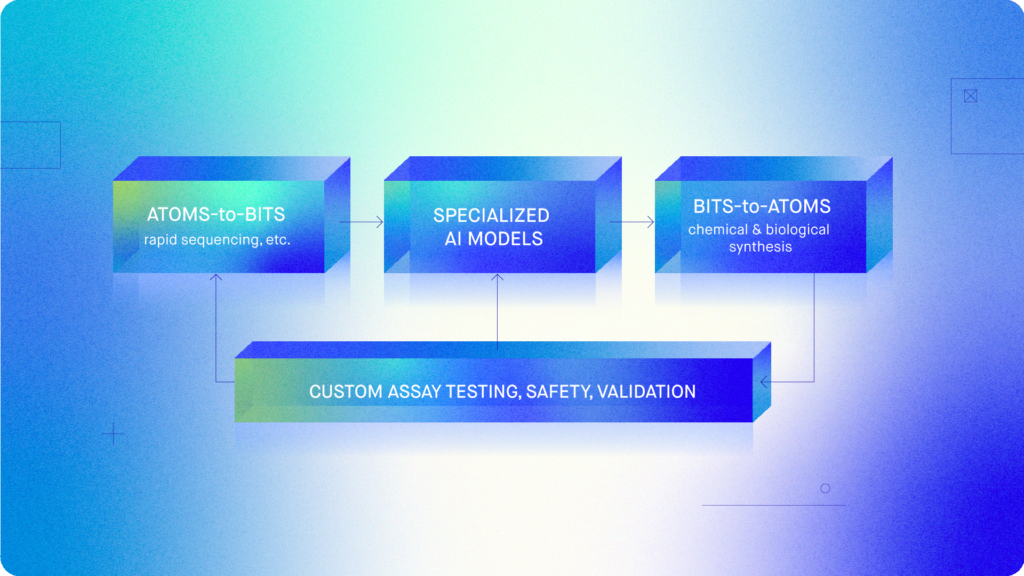
What’s more, we know from early iterations of generative science that the quality of scientific output is only as good as the quality of scientific input. The best results are based on accurate and well-curated data, and which are verifiable and reproducible, particularly when applied in sensitive areas like human biology. Noisy data or so-called data leakages not only produce unreliable and unreproducible results. They also undermine investment and trust in other areas of generative science that can transform industries and save lives.
AI SCIENCE IN ACTION
The centerpiece of generative science is combining AI-powered software with hardware that can accelerate the pace of experimentation and analysis. In 2009, researchers did this for the first time with a robot called Adam that predicted gaps in the known genome of bakers’ yeast. Now we have the tools to supercharge that process.
The next iterations will autonomously conduct the entire scientific process. Robotics systems will use AI to postulate hypotheses, conduct experiments with physical materials, analyze experimental data, and then use relevant learnings to formulate new hypotheses.
This robotic cycle doesn’t replace human creativity, but it does minimize human error. The same way developer tools empowered software engineers with new features and functionality, generative science will empower lab scientists to think expansively without the time-consuming and laborious work of measuring, moving, and observing materials.
AI-powered robotics will be especially powerful in drug discovery. TechBio company Recursion has developed an operating system that can perform millions of automated experiments every week to explore how biological systems and chemistry interact. Other companies are building platforms designed to autonomously discover synthetic chemicals and new materials. Accelerated lab research will yield broader possibilities in every field of science.
BUILDING CRUCIAL GUARDRAILS
Humans have always been the central conductors of science, and they will continue to be. While generative science can enhance our capabilities and speed up discovery, human oversight is key to keeping systems running safely and ethically. Trained scientists must design the experiments, interpret the results, and ensure that the technology can be accessed only by those who know how to manage it and protect it.
Such powerful technology poses the classic dual-use dilemma. Generative science technology that improves life on earth could be co-opted in the wrong human hands. The same biological printer that makes it possible to print food-grade plastic with no petroleum could also be used to print a bacteria ten times more lethal than E. coli. The same chemical synthesizing engine that makes the compound ingredients for a breakthrough battery could be directed to build devastating bombs and explosives.
That’s why for generative science to be world positive, it must work on the broad E-T-H-I-C-S checklist that I developed with my colleagues at Obvious.
The systems behind AI-powered science must be explainable and transparent to build trust in how systems process complex data to arrive at complex conclusions.
Their work must be human-centered and inclusive to minimize bias and blinders that could disadvantage broad communities, and they must promote civility and progress across the broader world. Crucially, these systems must be sustainable in how they consume energy and resources. While governments scramble to design regulatory frameworks for AI, we encourage founders to act now, staff their own red teams, and build safeguards and guardrails into their AI systems.
BRIGHT MINDS NEEDED
We believe generative science will create an exponential acceleration in scientific breakthroughs. The innovative startups that drive these breakthroughs will need cross-disciplinary teams that live at the intersection of AI and some of the world’s largest industries. We’ve highlighted some exciting examples of AI in climate, healthcare, and robotics. That’s just the beginning. We’re searching for generative science startups also reimagining future growth industries like robotics and advanced materials.
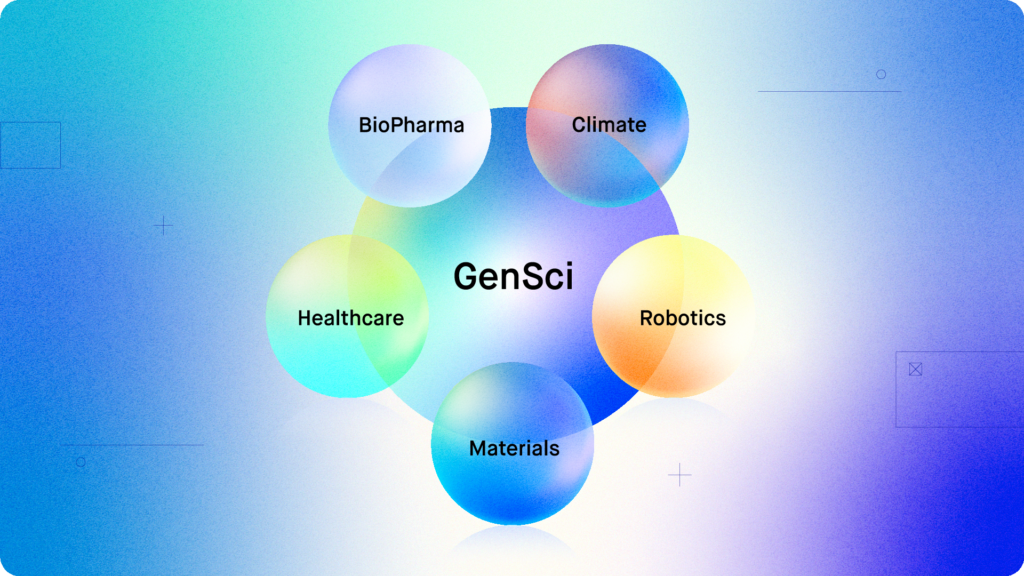
Obvious is investing at these frontier intersections, and we’re convinced that the crossroads of AI and biology will spur advances that transform planetary and human health. Overlaps of climate and AI will yield new solutions to accelerate renewable energy and mitigate climate change. Like any exponential technology, generative science will also bring new risks. We’ll need ethical, responsible companies to shepherd this technology in ways that dramatically improve human and planetary health.
In 1932, T.S. Eliot famously wrote, “Genuine poetry can communicate before it is understood.” Generative science is on a trajectory to become the greatest, most poetic creation of our species, communicating scientific breakthroughs that humans will subsequently test, validate, explain, and understand. The marriage of human ingenuity with generative AI represents a profound symbiosis, propelling us towards a future where the limits of what we can imagine and achieve in science are continually challenged and, to an increasing degree, surpassed.
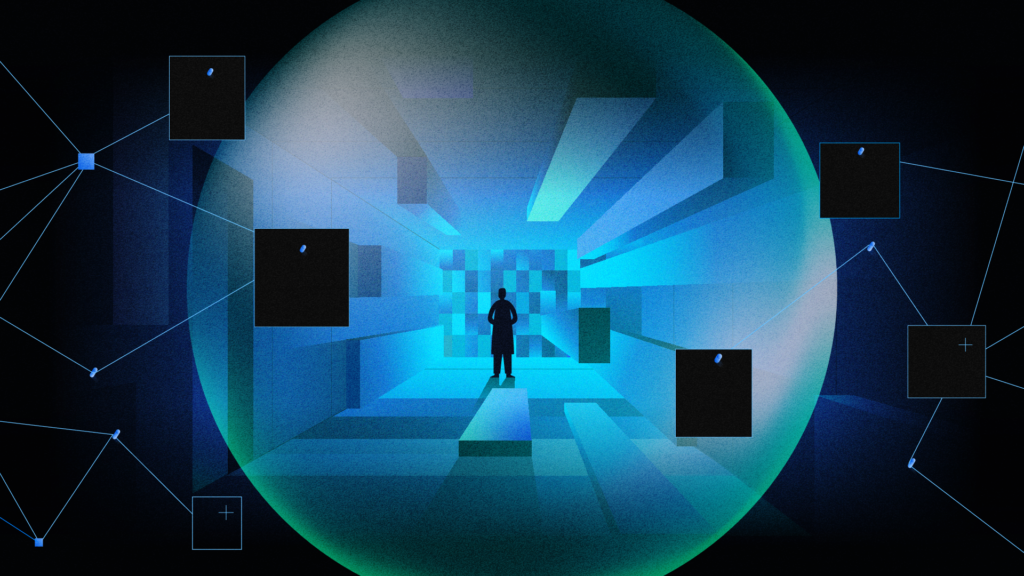