The E.T.H.I.C.S. checklist to sustain and grow AI responsibly
Kahini Shah |
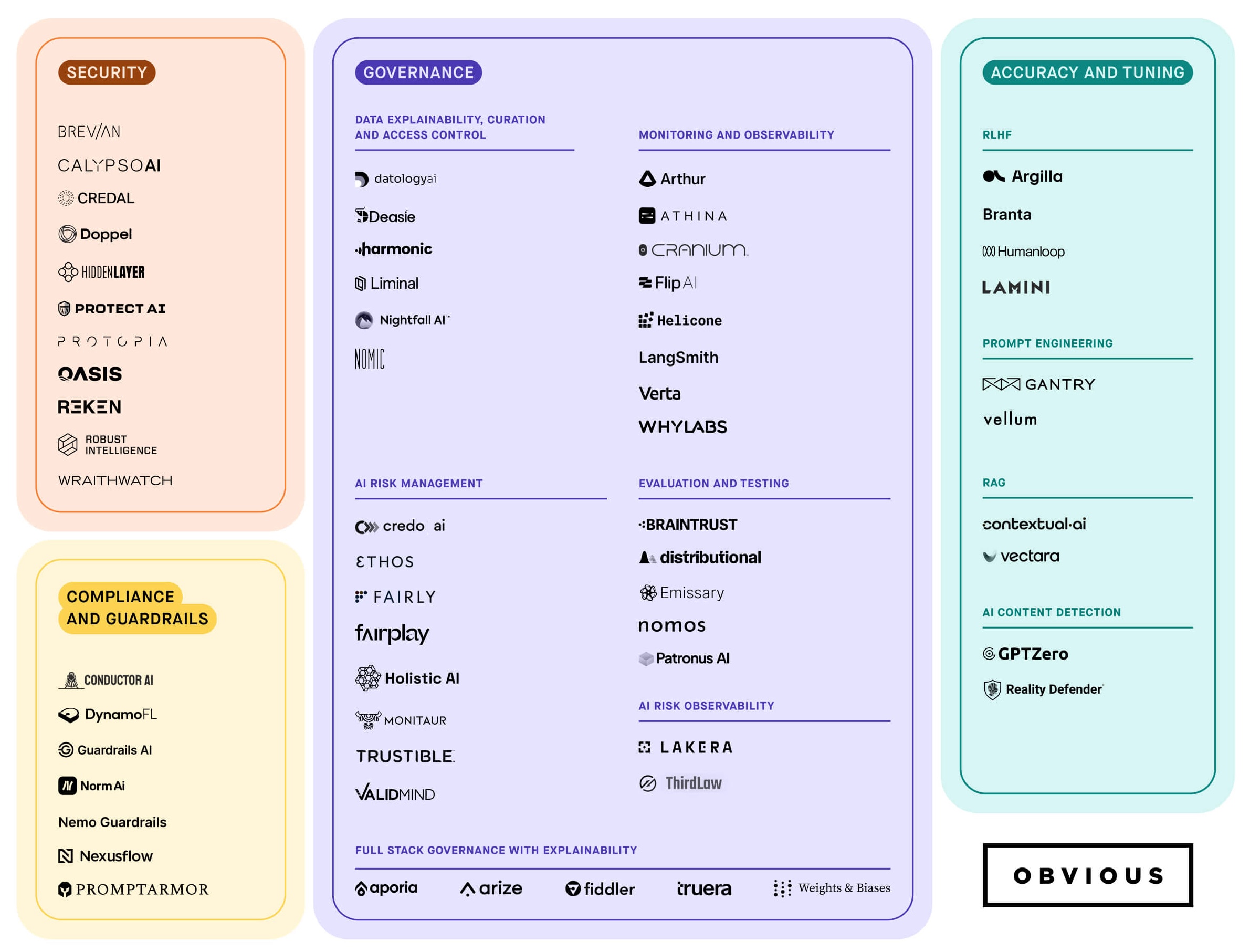
The age of generative AI is upon us, and it’s already proving to be a disruptive new technology. Generative AI has already shown how it can help researchers, entrepreneurs and corporate development teams do incredible things across the information and business economies.
The benefits are abundant. AI systems promise to transform health care by making better medical diagnoses and reducing administrative costs. AI will change how we work, increasing human creativity and helping people develop new skills. And it will promote human health and well-being with everything from better vaccines to personalized therapeutics.
But we also must consider the unintended side effects and risks. At Obvious, we encourage our companies to regularly ask, “What could possibly go wrong?” to build better safety, trust, and ethics into their technology.
Here’s our simple E.T.H.I.C.S. checklist of questions we ask to maximize the benefits of AI and mitigate what could go wrong.
Explainable
Are significant decisions made by your AI explainable? Can humans appeal, modify, and override those decisions?
Explainability in deep learning was already difficult, it’s even harder now with larger models. But explainability is crucial to understand how AI arrives at simple conclusions from complex data. For example, AI can use alternative data sources to make the credit underwriting process faster and fairer to marginalized economic groups, but it’s important to understand the models and benchmarks to verify that decisions are made correctly and fairly.
Frameworks, such as Stanford HELM, can help benchmark AI products and encourage greater transparency in training data curation and model development processes. Companies such as Arize allow you to monitor, troubleshoot, and evaluate your models, while companies like Patronus help detect LLM mistakes.
Transparent
Are your development, training datasets, and deployment processes transparent? Do you have a process to receive feedback and make changes from the broader community outside your team or company?
We’ve already seen the importance of transparent data in image and video generators. We don’t understand how many of these large models are trained and what data sets they draw from. Many image models have been trained on the LAION dataset, which was found to have explicit pictures of children. Authors, artists, and media companies have also raised copyright issues over the unauthorized use of their work.
Transparency can come in many forms. It’s important to understand the data a model is trained on, the techniques used, and how the model is weighted. Some systems, such as OpenAI, are closed. We are excited about the rise of open-source models like Llama-2 and Mistral AI, which are taking positive steps, like disclosing model weights.
Human-centered
Is your AI designed to meet human values and needs? Does it prioritize the well-being of people?
We can already see the possibility of deploying AI therapists or AI financial advisors. But when people interact with these systems, they need to be able to trust the AI is working in their best interest. That means outputs must not only be factual, but also compliant, legal, and safe.
Putting the well-being of humans front and center can start with smart government regulations, such as the European Union’s AI Act that prohibits systems that are subliminal, manipulative, or deceptive. It’s also an opportunity for companies to design responsible safeguards. There are potential solutions in early-stage companies like Guardrails AI and Norm AI that screen AI outputs for compliance, legal and ethical violations.
Inclusive
Have you taken steps to identify and remove bias from your AI systems? Are you testing your AI to ensure it does not inadvertently discriminate against any group of people?
AI models are only as good as the data they are trained on. If we train a model only based on mammals, we cannot ask it about snakes. In the same way, we must ensure that data for sensitive use cases like clinical medical decision support is inclusive of racial, ethnic, and economic groups so we can have confidence that outputs are free of bias.
Not every user—or in this example, every doctor—needs to be familiar with full data sets. But regulators and administrators can rely on infrastructure tools, such as Nomic that help users understand data sets, or Datalogy that helps curate, and catalog datasets.
Civil
Does your AI take steps to ensure civil communication? Does it drive conversation and action that is factually correct and beneficial to society?
We’ve seen ways AI can be civically harmful, such as when fake AI robocalls of President Biden attempted to suppress the vote, or when a fake image of Pope Francis in a casual puffy jacket was viewed by millions. Deceptions can have widespread consequences. Consumers lose nearly $9 billion every year in financial fraud, which experts expect AI to make worse.
We need solutions to help detect and protect users from deceptions big and small, but particularly the ones that can cause widespread social disruption. Companies like Reken are working to prevent AI-based cyber attacks by detecting deep fake content and autonomous fraud. Digital watermarking is another way companies can identify and label how content is made. And techniques such as Retrieval Augmentation Generation can help contextualize information and reduce hallucinations, a feature innate to LLMs.
Sustainable
Have you considered the environmental impact that your AI has on the planet? Is there a path to make your AI data center carbon neutral?
Every query to an AI model comes with an environmental cost. Creating one image with generative AI takes as much energy as charging your iPhone or driving your car four miles.
Making an AI data center carbon neutral will require continuous efforts, such as using renewable energy, investing in cooling systems and carbon offsetting, and monitoring and reporting emissions.
Companies are looking at new ways to power their data centers. Elementl is pursuing nuclear reactors and Zanskar is working to harness geothermal energy. Making an AI datacenter carbon neutral will require continuous efforts toward renewable energy, sustainable cooling systems and carbon offsetting, and monitoring and reporting emissions.
Are you building a world positive generative AI startup? Drop us a line at ai@obvious.com