How Generative AI Is Transforming Healthcare
Kahini Shah |
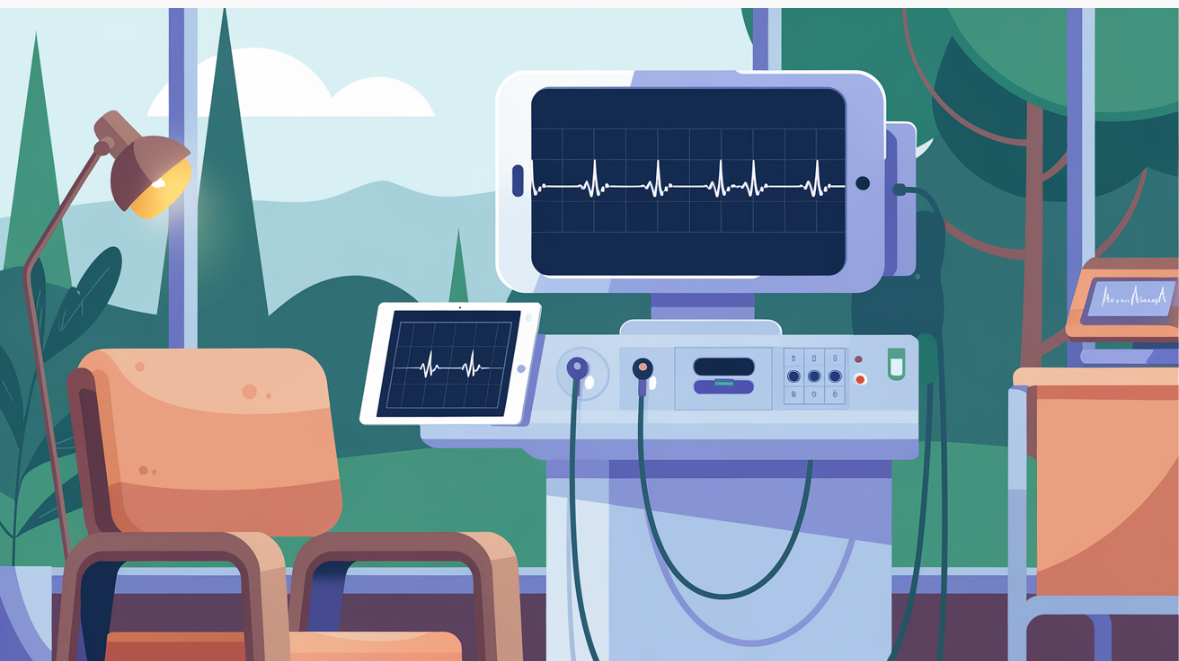
In healthcare, big leaps forward are on their way. In a world of complex health challenges, including rising chronic disease rates, doctor and nurse shortages, unequal access to quality care, and increasing aging populations, we see the biggest opportunities for AI-based transformation of healthcare in three key areas:
Administrative
Low-risk and low-complexity administrative tasks are most ripe for AI disruption.
Of the $4 trillion the U.S. spends yearly on healthcare, one quarter or $1 trillion goes to administration. These are tasks like scheduling appointments, managing paperwork and records, insurance verifications, and billing. This high cost diminishes quality care. If doctors spend 25% of their time on administrative tasks, that’s more than a day a week they’re not seeing patients, promoting lifestyle improvements, or making early diagnoses that could save lives.
Early innovators are working to save administrative time, cost, and efficiency. Obvious portfolio company GenHealth is improving a common administrative hurdle—prior authorization—using their large medical model. And Obvious has invested in an AI company currently in stealth that is pioneering agentive-based clinical management that eases burdens on physicians for mundane workflows.
What’s working: Language-based workflows like physician note-taking, coding, and prior authorization save doctors minutes per patient, adding up to hours per week.
What’s next: Bigger leaps in agentic workflows, including scheduling and referral management, are on the horizon, which will ease practice management. Advanced voice
capabilities will also improve patient experience, expediting tasks like confirming insurance coverage or scheduling time to talk with a doctor or nurse.
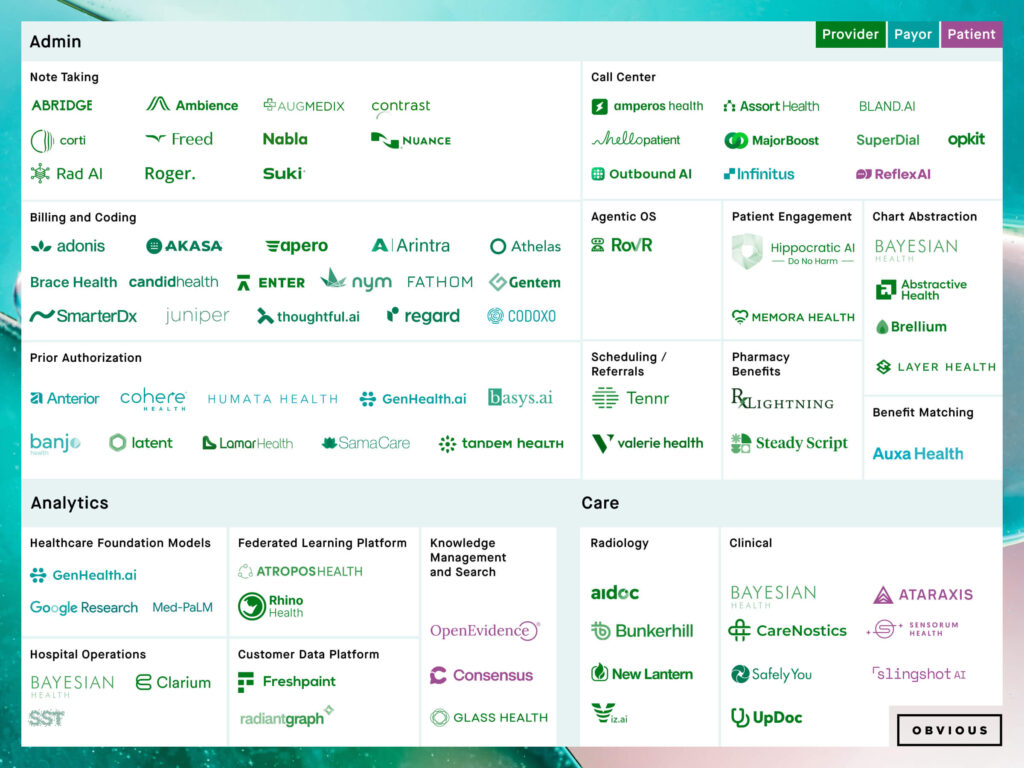
Analytics
Medium-risk and medium-complexity analytics will get a powerful boost from AI.
Abundant high-quality data is key to transforming healthcare from a reactive, sickness-care model to a proactive, preventative-care approach. When trained and properly calibrated, transformer-based AI can identify the statistical risks of various health incidents—like strokes, dementia, or cancer—months or even years earlier than via traditional methods. This allows for a clearer distinction between high and low-probability risks, enabling more targeted interventions. The result will not only be improved health outcomes but also significant cost reductions. Cardiac events, for example, cost the U.S. health system over $230 billion a year. Spotting warning signs and starting statins early can reduce events by as much as 25%.
Obvious portfolio company GenHealth is working on creating personal data insights by training a Large Medical Model (LLM) on medical data from 140 million patients.
What’s working: Current data-based systems help judge physician quality, patient outcomes, and operational efficiency. AI will soon enable legacy systems to ingest and analyze large unstructured data sets to create more holistic and accurate predictions.
What’s next: Combining data sets via multimodal models that can handle more data types to get better and more accurate predictions—across ages, ethnic groups, and even to individual patients—will lead to more personalized patient insights.
Clinical Care
Innovating clinical care is high risk and high complexity but holds the biggest potential to save and improve lives.
According to the U.S. Department of Health and Human Services, 5.7% of patients who visit the ER each year get a misdiagnosis. That translates to 7.4 million people misdiagnosed in an ER alone, to say nothing of errors in doctors’ offices, hospitals, and community clinics. Nearly half of those 7.4 million suffer an adverse event from their misdiagnoses, and 1 in 20 suffer serious harm.
AI can substantially improve diagnostic accuracy and clinical care and help make better and more informed decisions. Obvious portfolio company Ataraxis is working to optimize diagnoses and treatments for breast cancer based on pathology data and patient-specific biological markers. Another portfolio company, currently unannounced, is a platform to create, validate, deploy, FDA clear and monitor in production clinical and operational AI solutions.
What’s working: Radiology is one of the first clinical specialties to see mature AI technology that can improve image analysis and reduce diagnostic errors.
What’s next: Multi-modal clinical augmentation, in which co-pilot assistants advise and guide doctors in real-time, will be game-changing. These systems will incorporate up-to-date medical research that clinicians can apply to a patient’s broader modal data, including scans, images, and biometric data, to facilitate more accurate diagnoses and better outcomes.
We believe that strong datasets and smart algorithms will form centers for excellence, where collective learnings and AI insights will be stored together and be made available to physicians in moments of need as they treat patients and uplevel patient care.
We’re optimistic about AI-based innovation in these areas. If you’re working in any of these fields, please get in touch.