Getting Physical: The New AI Frontier of Robotics
Kahini Shah |
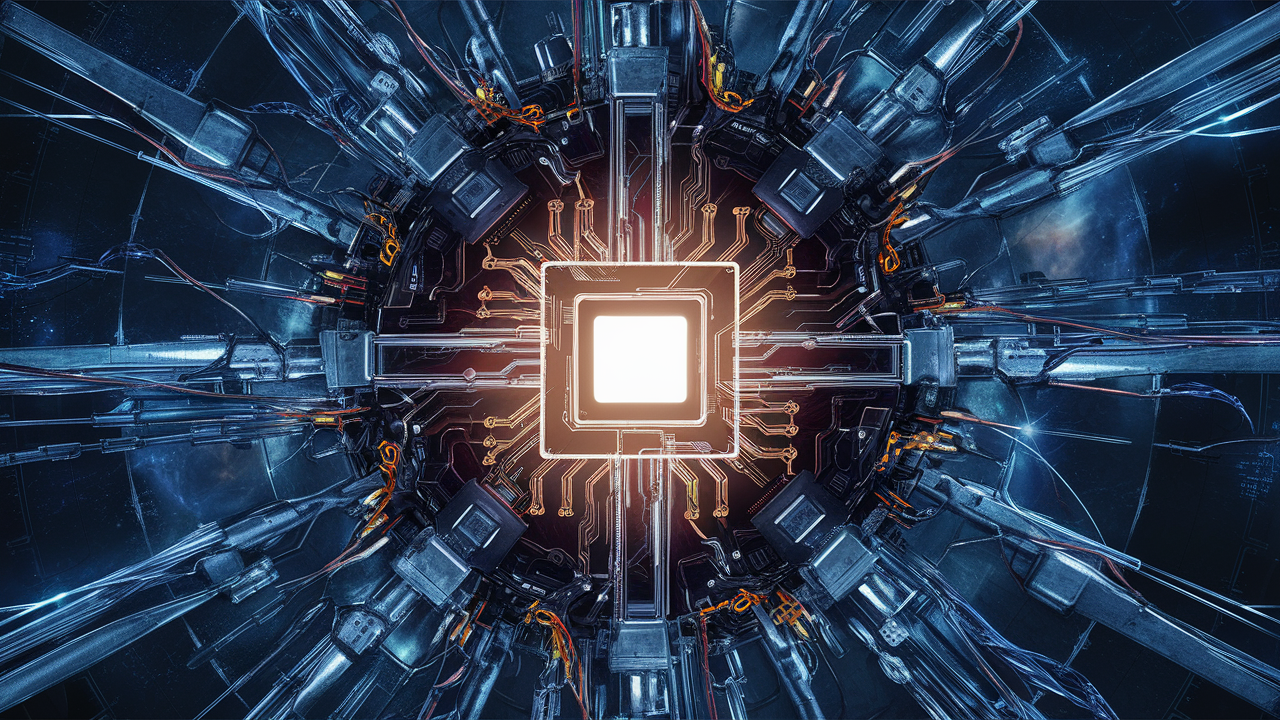
With their recent releases, OpenAI and Google have shown the profound capabilities of AI systems that can read, write, see, and even understand. But even the best generative AI web applications can’t interact with the physical world. Robotics are the next frontier of generative AI that will bridge that gap, creating what visionaries like cognitive scientist Gary Marcus and Apple cofounder Stephen Wozniak consider the definition of artificial general intelligence.
For years, robots have grown in market scale and capabilities. Early systems moved items at grocery counters and automatically opened doors. More advanced systems have been deployed in warehouses to support e-commerce processing, like those used by Kiva Systems, which Amazon acquired for $775 million in 2012, its second-largest acquisition at the time. Robotic vacuum cleaners from iRobot (market cap: $300 million) and NASA’s Mars rovers navigate unstructured environments with simultaneous localization and mapping (SLAM) technology, while self-driving cars produced by Waymo (valuation: $30 billion) use convolutional neural networks to understand stop signs and lane markers.
But these robotic systems and companies face two distinct problems: the world is filled with infinite uncertainty and detail, and the need for tightly coupled software hardware yields limited abilities.
Pre-transformer neural networks are adaptable but they are also fragile. They require tens of thousands of training samples to perform tasks like placing different foods into a container. Similar issues arise when comparing learning a policy to explicitly encoding one. For instance, if a system needs to handle and pack strawberries but has never seen them before, there’s a high chance it will squash the strawberries. The interfacing of hardware and software has also traditionally favored simplicity. The robotic stack combines perception systems (cameras, radar, lidar, pressure sensors) with control systems (motors, actuators). These systems must work harmoniously, leading to tightly integrated hardware and software. High-performing systems, like the robotic dogs and bipeds from Boston Dynamics, are typically very expensive. Those without the expertise and resources must opt for cheaper, less capable alternatives.
A new paradigm is emerging with breakthroughs that are making robotics more versatile and task-agnostic. ChatGPT works by predicting the next word or pixel. Robotics can use a similar architecture to predict the next action.
In theory, a foundational model of robotics or a robotic brain can perform a variety of tasks and learn a new task with more limited training samples. The Roomba is excellent at vacuuming. Companies like, like Figure x OpenAI promise systems that can not only vacuum but can also put away dishes and maybe even cook a meal.
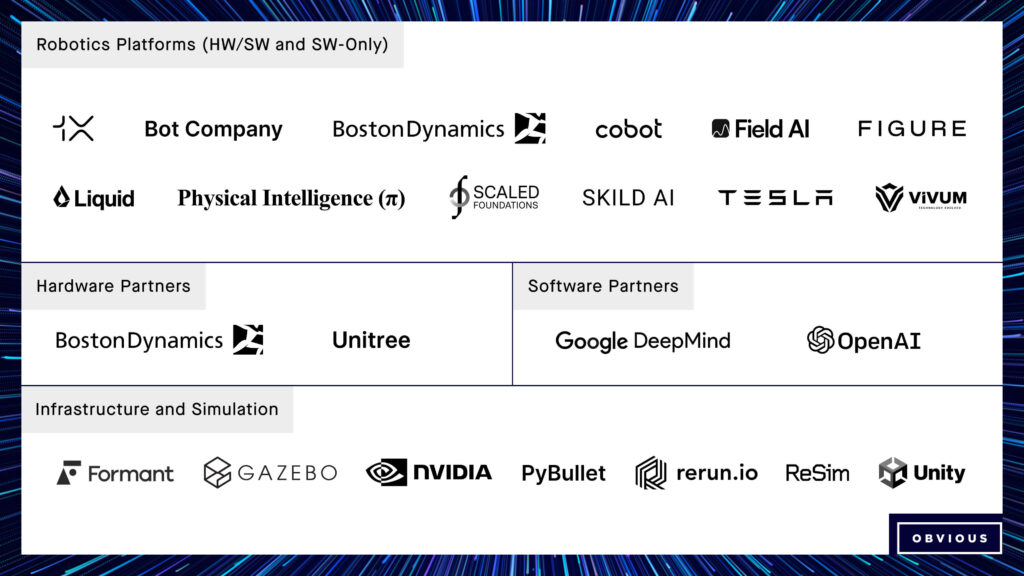
New techniques are incorporating transformer-based architecture to inform complex actions. DeepMind’s Robotic Transformer 2 (RT-2) has shown it can learn from combining the web and robot data to perform complex tasks. Carnegie Mellon Professor Deepak Pathak has demonstrated how perception and control can be combined. One prototype used only optical information from a camera image to effectively perform parkour, navigating obstacles it had never seen before, cheaper, less performant hardware.
Breakthroughs in architecture are aided by advanced training and policy learning techniques aimed at more generalizability. Physics-based simulations like Nvidia’s Isaac Gym can teach robots skills such as walking in a simulated world, and offline reinforcement learning can make them more robust. Berkeley professor Sergey Levine has demonstrated powerful learning pathways through imitation learning and behavioral cloning using teleoperation data.
Looking forward, innovations in neuro-symbolic systems blend logic-based rules with neural networks, offering more deterministic reasoning. Liquid networks, a technology pioneered by Ramin Hasani, promisesgreater explainability and adaptability.
This is an exciting space with major tech players like Google, Nvidia, Tesla, and possibly Apple unveiling new robotics technology, and startups like Figure, SkildAI, 1X, and Physical Intelligence are raising hundreds of millions of dollars. These companies are pursuing different strategies toward the same goal of making robotics more versatile. They differ in algorithmic approaches for generalized intelligence, modes of hardware and software integration, robotic form factors, such as humanoid, quadruped, or wheeled, and system priority, either dexterity or navigation.
Compared to the rest of the robotics market, companies pursuing these breakthroughs are raising funds at astonishing valuations, indicating high market potential. Nascent start-up Physical Intelligence is valued at $400 million, SkildAI is thought to be valued at $1.5 billion, and Figure AI is valued at $2.6 billion. Bain estimates that the robotics market will grow from $40 billion to as high as $260 billion by 2030. Yet, with transformative advances in versatile, user-friendly, and robust systems, we may see much higher growth.
We’re optimistic about these new frontiers in robotics. And we’re excited about the companies building the AI-powered robots of the future.
If you’re working in this space, please get in touch at kahini@obviousventures.com